Information, uncertainty, and cognitive biases: we’re all irrational in predictable ways
Even while I was preparing for the field expedition to Svalbard, post-polar adventure and crashing back down to earth, other work carried on. In fact, while learning more about trust in big data for ocean decision-making, specifically the case of Calanus finmarchicus, I’ve talked to some interesting folks. They are experts in:
- Calanus, or plankton generally,
- fishing for Calanus,
- technology that can be used to monitor and understand Calanus,
- how data and information are used to manage Calanus, or
- some combination of the above.
In short, a bunch of smart people who know a lot about things that I don’t know a lot about.

I asked them about their work, and how it relates to understanding, managing, or catching Calanus. I asked them about the kinds of data and information they use, and how they think about big data. I asked them about how they think data and information connect to making decisions about Calanus fishing and management, and what role they play in making those connections. And I asked them about trust, and how they think about it – for themselves personally, in their work, in data and information, in decision-making processes.
One thing stands out from the conversations I’ve had: uncertainty in science and society. The researchers I’ve spoken with are painfully aware of the limitations of the methods they use and the data they work with. Being uniformly highly trained experts, using cutting-edge technologies, they will tell you, at great length, about what they don’t know, what can go wrong, and how they may or may not catch any errors. In short, they’re obsessed with uncertainty.
Uncertainty and the science of cognitive biases
In society, uncertainty is a funny thing. Humans appear to be hard-wired to be uncomfortable with uncertainty and to look for ways to resolve it. We are, all of us, subject to cognitive biases, or predictable departures from purely rational behavior, that can affect our judgments when we face uncertainty. Here are three examples drawn from psychologist Daniel Kahneman’s book Thinking, Fast and Slow.
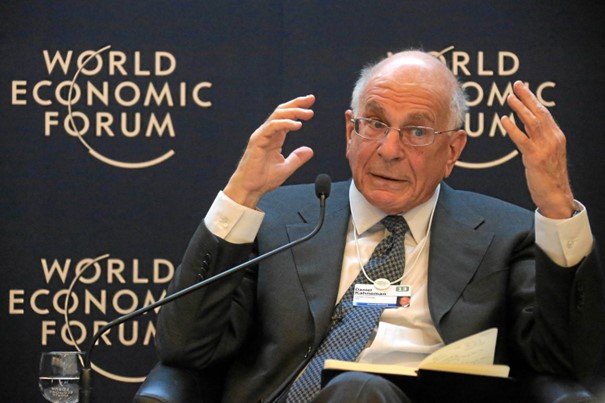
One is anchoring bias, whereby a number affects the estimation of an uncertain value. In an experiment Kahneman conducted with Amos Tversky, they provided some people with a ‘random’ low number and others with a ‘random’ high number, and then asked participants to estimate an unrelated value (the percentage of African nations in the UN). People with a low number gave a significantly lower estimate than those with a high number, though, rationally, the ‘random’ spin of a wheel has nothing to do with African nations’ UN presence. Faced with uncertainty, our brains want a reference point.
See previous blogs by Kate Crosman
Moving to Trondheim to work with Big Ocean Data by Kate Crosman
How tiny zooplankton can help us understand trust in Big Ocean Data by Kate Crosman
Home of the northernmost magic by Kate Crosman
Another example is the availability heuristic. Sometimes it’s easy to think of examples of an event because it happens frequently – it seemed like it rained a lot in Trondheim last fall because there were a lot of rainy days in Trondheim last fall. This is what makes this a heuristic (a rule of thumb) rather than just a bias. But other things— personal experience, media attention, recency — can also make us overestimate frequency simply because it’s easier to bring an example to mind. That’s where the availability heuristic slides into a bias.
A third example is loss aversion. We tend to negatively value our losses more than we positively value equivalent gains. In short, we lose more satisfaction from losing 500 NOK than we gain from winning 500 NOK. This bias holds equally true for potential losses.
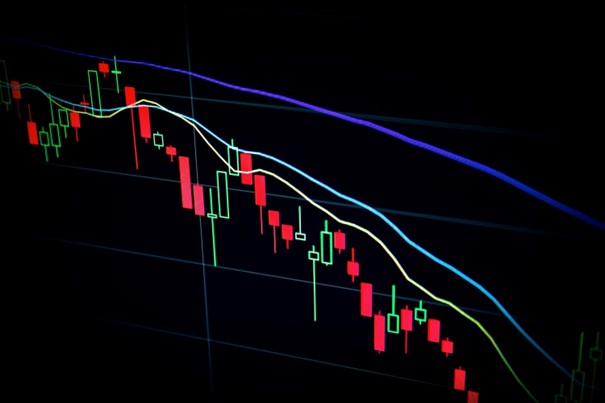
As these experiments have mostly involved participants from Western, educated, industrialized, rich, democratic (WEIRD) populations, we’re not yet entirely sure whether these cognitive biases affect all kinds of people equally. The scientists I’ve spoken with are WEIRD, too (hmm…), but they’re also trained to think hyper-critically about data and information, using systematic approaches and rigorous tools. Indeed, much scientific training focuses on understanding, categorizing, and quantifying uncertainty so that the bounds of what research can tell us are clearly defined.
The problem is that scientific uncertainty is sometimes communicated in ways that can be misunderstood by people who aren’t scientifically trained. Communicating scientific uncertainty also does little to address the ways that people hear and receive information in non-scientific contexts: filtered through cognitive biases.
So, what does this mean for trust in big data for Calanus?
Well, if we want people to trust our data – we need to understand how those decisions are likely to be perceived by the people they affect. Take the example of opposition to the coastal Calanus fishery from cod and herring fishers, who worry about bycatch of fish larvae in Calanus nets. How is a recent proposal to increase the coastal Calanus quota by up to five times likely to be perceived? Anchoring bias is likely to make that increase especially concerning. Resistance may be strongest where cod and herring fishers can directly observe Calanus boats out on the water, thanks to availability bias. And resistance is likely to be reinforced by loss aversion, as we weigh even uncertain losses especially heavily.
Can different kinds of data and information regimes influence such resistance? I’m hoping to learn more about exactly that in the upcoming stage of my research – stay tuned!
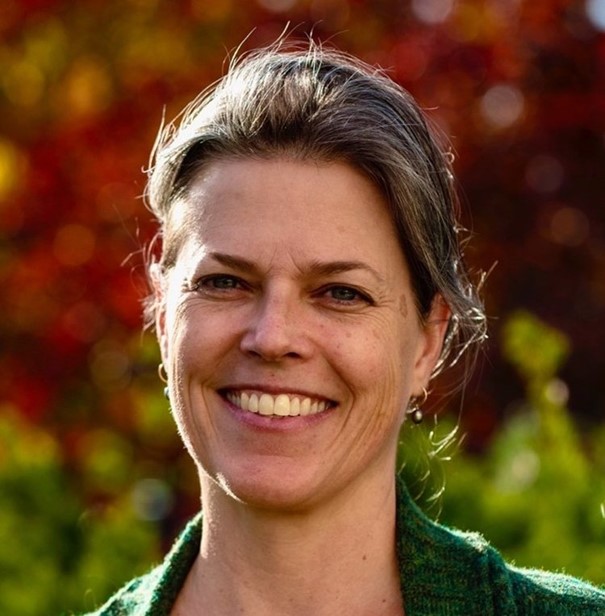
Kate Crosman
Kate Crosman is a social scientist with expertise in the complex governance of oceans and coasts doing a post doc at the Department of Marine Technology at NTNU and NTNU AMOS. She’s spending 3 years in Norway as an André Hoffmann Fellow in Big Ocean Data, studying trustworthiness and trust in big data for ocean decision-making. In this blog, Kate is writing about cognitive biases associated with processing information, and how they might influence the fishery for Calanus finmarchicus – a tiny plankton species that became a starting point for her research.
Search
Søk
Categories
- Arctic Research
- Arkitektur
- Bærekraft
- Bioingeniørfag
- Biologi
- Biology
- Biomedical Laboratory Science
- Biotechnology
- Bioteknologi
- Chemical Engineering
- Chemistry
- Climate
- Computer Science
- Datateknologi
- Digital
- Elektronikk
- Energi
- Energi
- Energy
- Engineering
- Engineering
- Environment
- Food Science
- Forskning
- Fysikk
- Fysikk
- Havbruk
- Informasjonsteknologi
- Informasjonsteknologi
- Ingeniørvitenskap
- Kjemi
- Kjemisk prosessteknologi
- Kjemisk prosessteknologi
- Kreftbehandling
- Kybernetikk
- Marine Technology
- Materialer
- Materials Science
- Materialteknologi
- Matvitenskap
- Meninger
- Miljø
- Min ph.d.
- My PhD
- My PhD
- My postdoc
- Nanotechnology
- Nanoteknologi
- Ocean
- Oil and gas
- Physics
- Research
- Simulering og visualisering
- Spør en forsker
- Studentliv
- Sustainability
- Ukategorisert
- Universitetsliv
- University Life
Kategorier
- Arctic Research
- Arkitektur
- Bærekraft
- Bioingeniørfag
- Biologi
- Biology
- Biomedical Laboratory Science
- Biotechnology
- Bioteknologi
- Chemical Engineering
- Chemistry
- Climate
- Computer Science
- Datateknologi
- Digital
- Elektronikk
- Energi
- Energi
- Energy
- Engineering
- Engineering
- Environment
- Food Science
- Forskning
- Fysikk
- Fysikk
- Havbruk
- Informasjonsteknologi
- Informasjonsteknologi
- Ingeniørvitenskap
- Kjemi
- Kjemisk prosessteknologi
- Kjemisk prosessteknologi
- Kreftbehandling
- Kybernetikk
- Marine Technology
- Materialer
- Materials Science
- Materialteknologi
- Matvitenskap
- Meninger
- Miljø
- Min ph.d.
- My PhD
- My PhD
- My postdoc
- Nanotechnology
- Nanoteknologi
- Ocean
- Oil and gas
- Physics
- Research
- Simulering og visualisering
- Spør en forsker
- Studentliv
- Sustainability
- Ukategorisert
- Universitetsliv
- University Life