Could artificial intelligence improve our treatment of COPD (kols)?
Chronic obstructive pulmonary disease (COPD) is one of the leading causes of death, both in Norway and globally. It is caused by inflammation and scarring of the airways, leading to chronic cough, difficulty breathing, and in many cases destruction of the surrounding lung tissue. Imagine a tree upside down, the airpipe or trachea is the main stem, and then the airways branch out, ending in small twigs. In our lungs these small twigs are called the distal or peripheral airways, and it’s these distal airways that are affected by COPD.
Causes – more complicated than smoking
It is well-known that smoking is an important cause behind COPD. However, only 20% of those smoking gets COPD, and as many as 20% of those with COPD have never smoked! In other words: This is more complicated than just smoking. We know that several other factors also influence the development and progression of COPD, including genes, environmental factors like air pollution, events before birth or during childhood, and concurrent diseases, so-called comorbidities.
Mapping common comorbidities
Our project aimed to map common comorbidities in persons with COPD and look at how they affect important outcomes. These outcomes were death and frequency of hospital admission due to severe worsenings (exacerbations) of COPD. We included a little more than 2000 participants with COPD from the HUNT Study, a large population-based study from the northern part of Trøndelag. We identified ten different comorbidities among these participants: anxiety, depression, osteoporosis, heart infarction, kidney failure, diabetes, high cholesterol, over- and underweight and hypertension.
Artificial intelligence
When you have a large data set with many different data points, it is often difficult to see meaningful patterns. Therefore, we used artificial intelligence, so-called machine learning, to do cluster analysis. A cluster is a group of similar things positioned or occurring closely together. This special type of cluster analysis is called a self-organizing map. It is easier to understand if you pretend that you place the 2000 persons with COPD on a football field. Each person has a red and a blue flag. If you ask them to raise their red flag if they have diabetes, and their blue flag if they don’t have diabetes, and then take a picture from above, you will have a map with red and blue areas, indicating how diabetes is distributed in the crowd. If they are not allowed to change their position in the football field, and you ask them to raise their flags for each of the ten diseases, you get the map that you see in the figure below.
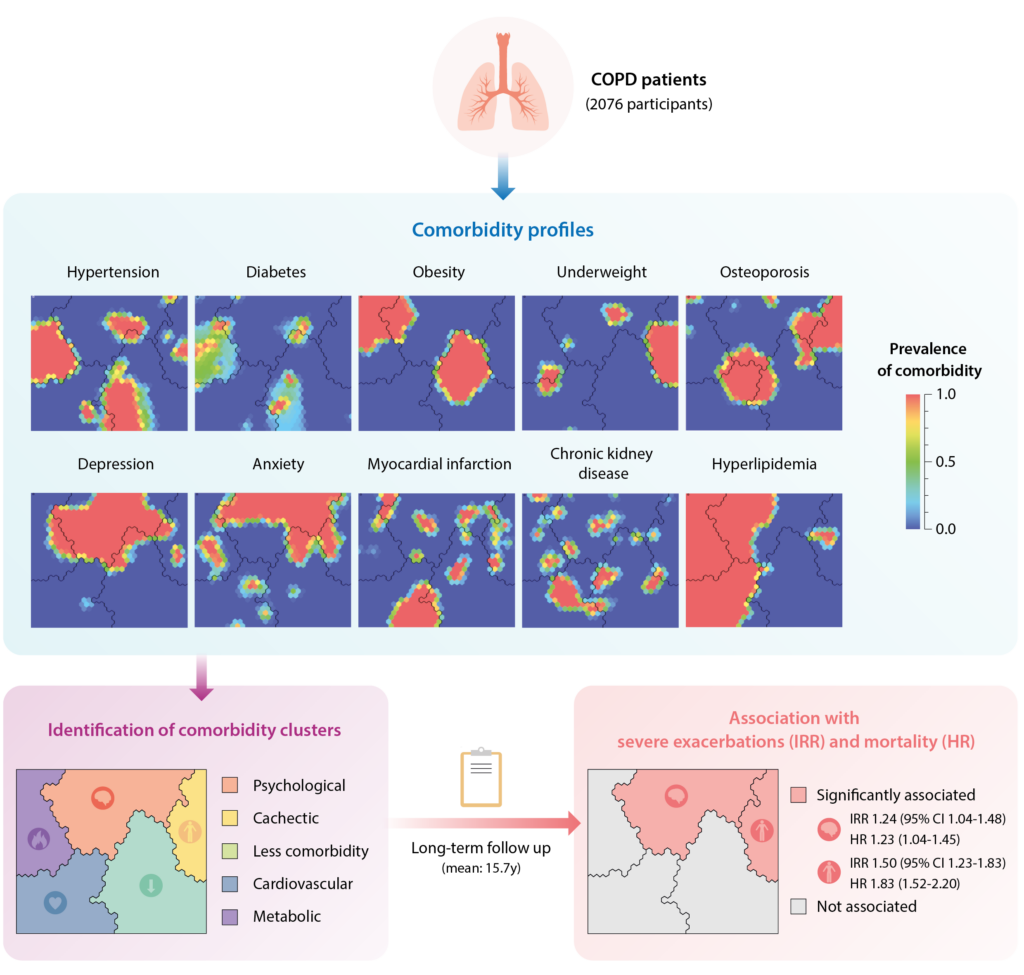
And here it gets exciting: you realize that many of the persons seem to have the same diseases (they are on the same position on the football field). The diseases are clustering together. For example, you can see that people with anxiety also have depression (the red color is concentrated in the same area of the anxiety and depression maps). And you can see that people with osteoporosis also tend to have low body weight. Doing this, we identified five different so-called comorbidity clusters. The ones mentioned above we decided to call the “psychological” and the “cachectic” clusters (cachectic is Greek and is combined by “kakos” and “hexis”, meaning “poor physical state”). We found that people belonging to these clusters had higher mortality (died earlier that those not belonging to these clusters) and were more frequently admitted to the hospital due to severe COPD worsenings.
Early treatment
It seems that clinicians need to be extra aware of persons who have both COPD and comorbidities like anxiety, depression or osteoporosis. Would early treatment of these comorbidities lead to better outcomes for these individuals?
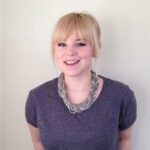
Sigrid Anna Aalberg Vikjord
Vikjord is a resident physician in respiratory medicine at Levanger hospital. She is currently working as a researcher at HUNT Research Centre, where she is affiliated with the lung and osteoporosis study.
- This author does not have any more posts.