Improving ultrasound images of the heart’s blood vessels
Coronary heart disease is a condition where the heart muscle (myocardium) does not receive enough oxygen and nutrients due to obstruction of blood flow in the heart’s blood vessels, known as the coronary arteries. It is therefore important to investigate the blood flow in these vessels. However, it is challenging to obtain accurate measurements due to the combination of poor blood flow, the constant movement of the heart throughout the cardiac cycle, and surrounding tissue causing noise signals.
Here at the Centre for Innovative Ultrasound Solutions (CIUS), we work on filtering away the noise signal generated from the tissue, and to automate this filtering process.
In order to achieve this, we are using certain components of the ultrasound signals called eigenvectors, which have shown to be very promising for blood and clutter separation. From the theory, we know that the eigenvectors with highest power are those containing tissue signal, so we sort the eigenvectors by descending power and we remove the first set of eigenvectors.
Here the challenge begins: How do we select which eigenvectors to remove? One option is to do it manually, which means looking at the 3D coronary images when removing different numbers of eigenvectors and deciding which is best. It would be better, however, to automate the process of selecting the appropriate eigenvectors.
In Fig. 1 you can see 2D projections of 3D coronary images using a standard high cut-off filter. The coronary is displayed in middle diastole (relaxation) which is the part of the cardiac cycle where the coronary vessel is most visible. During systole (contraction) there is almost no flow in the coronary arteries. Our goal is to remove flashing artifacts in early and late diastole in order to be able to detect blood flow. At these time points, tissue signal might be highly accelerated, which makes clutter suppression challenging.
Fig. 2 shows 3D coronary images at different time points of the cardiac cycle using a manual filter based on eigenvectors. In panels b) and d) there is still some tissue signal left but in a) and c) the coronary (blue circle) can be more easily identified.
In the future, we will investigate whether varying parameters such as increasing the frame rate could help to improve clutter filtering. It might also be interesting to examine coronary arteries situated in different locations. In general, we think that we are on the right way and eigenvectors represent the key to solve the clutter filtering challenge! Eigen-based filters are indeed able to adapt to the different phases of the cardiac cycle, while the conventional filters do not vary over time.
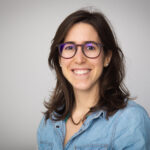
Cristiana Golfetto
Research Scientist, SINTEF, 2020-
PhD Candidate CIUS, NTNU 2016-2020
- This author does not have any more posts.